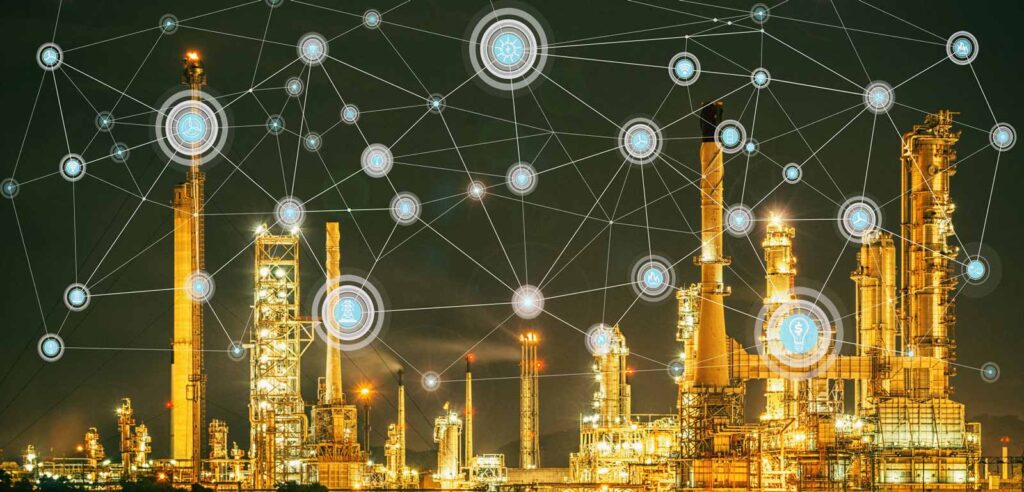
One of the main challenges in the oil refining and petrochemical industries is to continue operating while maintaining production equipment. When a plant is forced to shut down due to equipment failure or human error, the loss is tremendous. Therefore, there is strong demand for technology to predict equipment failure. Once stable operation is achieved, the next goal is to improve productivity and maximize profits. Since huge amounts of energy are consumed for separation and other processes in the oil refining and petrochemical industries, it is also important to reduce energy consumption.
Predictive Maintenance
Production equipment in the oil refining and petrochemical industries is regularly checked during scheduled maintenance. However, various parts of compressors, pumps, and other rotating equipment tend to wear out and deteriorate more quickly than expected due to fluctuations in the quality of fluid components and environmental conditions. This may lead to earlier performance deterioration or failure. Unexpected plant shutdowns cause enormous damage, so it is crucial to predict failures and take measures in advance. Quick planning and implementing a new or additional maintenance plan can minimize production losses. Anomalies can be detected not only by direct means
such as conventional vibration meters and ammeters but also by monitoring the process state. For this purpose, machine learning can be used to detect changes in the relation among various process variables compared with that during normal operation. Since there are various types of abnormality, it is not always possible to detect them based on the past relation among process variables. Therefore, AI-based forecasting models are also required to predict future problems.
It is necessary not only to detect signs of equipment failure but also to investigate and eliminate the causes for future operation. Performance degradation due to contamination in compressors, leakage due to corrosion of piping and tanks, clogging of equipment due to accumulated rust and polymerization, and other problems must be detected early. It is also necessary to identify the root cause. This is typically done by analyzing differences between normal and abnormal operations as well as between when equipment is deteriorated and when it is in the normal condition. To identify the root cause, a hypothesis is made and then verified using process data. When sufficient data on abnormal conditions are available, a classifier is created by machine learning to determine whether the current state is normal or abnormal. By analyzing the classifier model it is possible to determine which process variable contributes more to the current state.
Product Quality Prediction and Optimization
Product quality metrics are often not available in real time because sampling is performed and analyzed in a lab. For example, product quality may vary due to fluctuations in the quality of raw materials and environmental conditions, and it takes significant time to obtain lab results. Therefore, a model to predict product quality in real time based on process data is required. With multiple process variable inputs, this prediction model outputs the predicted value of product quality. This model is usually a nonlinear machine learning model based on actual data.
Quality problems are critical in petrochemical products, and in particular, batch products. Since there is a trade-off relation, it is often difficult to improve the quality and quantity of products simultaneously. Even if the production volume is reduced to improve the product quality, the product quality may vary due to fluctuations in the quality of raw materials, changes in environmental conditions, and other reasons, resulting in a certain percentage of defective products. Since poor product quality can occur due to a number of causes, it is often difficult to determine the factors. Thus, the causes of defective products must be analyzed.
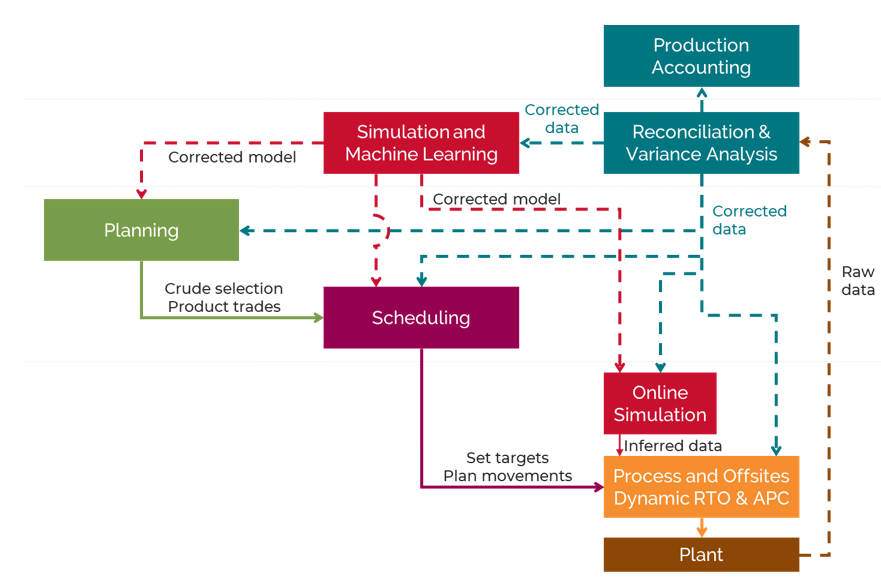
Today’s refinery optimization processes are highly manual and dependent on skilled staff with a strong knowledge of refinery processes and the relevant optimization technology. These staff are increasingly rare. Other challenges of refinery optimization today include:
Linear models are often used and have a limited range of validity
Maintaining the process simulation, production planning and production scheduling models is time-consuming and requires significant expertise
The data reconciliation process is largely heuristic and simplistic
Data is in silos and difficult to collect and manage
The key constraints are not adequately identified and challenged
Advanced control and real-time optimization strategies are not updated with plan and schedule changes
Schedulers focus on finding a feasible solution with limited time to minimize the deviation to the plan or optimize the schedule
The integration between the various applications is limited
Our planning and scheduling optimization models will invoke machine learning and/or cognitive computing functions, using live data, to deliver a step change in refining optimization. The benefits of this include:
-
Automated data driven optimization using AI
-
Intelligent, automated work processes
-
Automated data management and application integration
-
Cloud/On-Premise deployment to facilitate collaboration, scalability and rapid development and delivery of enhancements
-
Automated surfacing of optimization opportunities
-
Automated backcasting to close the gap between the plan, schedule, actual and optimum
-
Automated model updates to create a self-learning digital twin
-
Automated and optimized production scheduling
-
Multi-unit dynamic optimization