
According the International Society of Automation, a typical factory loses between 5% and 20% of its manufacturing capacity due to downtime. Traditional preventive maintenance processes require machines to be repaired at intervals based on time or usage. These methods, however, still result in significant instances of equipment failure resulting in idle workers, increased scrap rates, lost revenues and angry customers. In addition, preventive maintenance may replace parts that still have significant working life, which can be a waste of time and money.
Predictive maintenance is a technique that uses condition-monitoring sensors and Machine Learning or rules based algorithms to track the performance of equipment during normal operation and detect possible defects before they result in failure. Predictive Maintenance enables the reduction of both schedule-based maintenance and unplanned reactive maintenance by triggering maintenance calls based on the actual status of the equipment. IoT relies on Predictive Maintenance sensors to capture information, make sense of it, and identify any areas that need attention. Some examples of using Predictive Maintenance and Predictive Maintenance sensors include vibration analysis, oil analysis, thermal imaging, and equipment observation.
AI based predictive maintenance uses a variety of data from IoT sensors imbedded in equipment, data from manufacturing operations, environmental data, and more to determine which components should be replaced before they break down. AI models can look for patterns in data that indicate failure modes for specific components or generate more accurate predictions of the lifespan for a component given environmental conditions. When specific failure signals are observed, or component aging criteria are met, the components can then be replaced during scheduled maintenance windows. McKinsey and Company found that AI based predictive maintenance typically generates a 10% reduction in annual maintenance costs, up to a 25% downtime reduction and a 25% reduction in inspections costs.
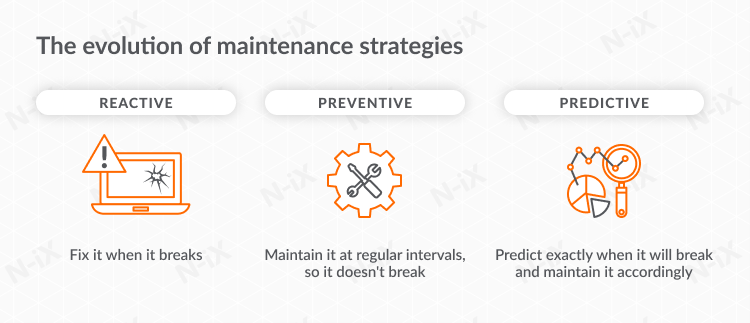
Predictive Maintenance vs. Preventative Maintenance
People often confuse predictive and preventative maintenance, however, there are significant differences between the two.
Preventive maintenance occurs at regular intervals based on the machine’s lifecycle, regardless of usage, to ensure that no issues emerge. For preventative maintenance, the only variable used to predict failure is the span of time since the previous maintenance was conducted. Regularly scheduled oil changes for your car are a familiar example of preventative maintenance. While preventative maintenance is a huge improvement over reactive service, it has its limitations. Essentially, it is using a very simple data model (e.g., car engines experience long-term problems if oil isn’t changed every 5,000 miles of travel), to make a very broad recommendation (e.g., you should bring your vehicle in ASAP for an oil change). A preventative maintenance model doesn’t factor in any conditions that are unique to your car’s engine, or how the vehicle has been driven.
Predictive maintenance continuously analyzes the condition of connected assets and equipment. It can collect multiple types of data to build detailed models that reflect equipment status, and how that equipment is being used. This way, data produced during the machine’s normal operations is analyzed and to propose much more accurate maintenance recommendations. This data is analyzed to reduce the probability of failures, and provides a richer understanding of the causes, likelihood, and time-to-failure if an asset remains without service. Unlike preventative maintenance, which consists of blanket rules, predictive maintenance delivers accurate and specific recommendations that reflect your equipment and how you’re using it. This accuracy also prevents unnecessary maintenance activities that can incur costs and downtime.
By applying the transformative combination of IIoT and AI to build very robust predictive maintenance data models, organizations are experiencing decreased downtime, greater work productivity, reduced field service costs, improved product design, and heightened worker safety.

Business Viewpoint
How does Predictive Maintenance impact an organization’s performance?
Predictive maintenance is often the first use case that is prioritized, and it is seldom the end game. In manufacturing, enabling predictive maintenance provides near term value by improving OEE and reducing maintenance costs. In the longer term, it enables data collection that supports more advanced use cases such as lot size of one or more effective or flexible production line configurations.
In machine building, any time it is proactive rather than reactive, there is an opportunity for value creation- for example, reducing truck rolls (sending out a truck for maintenance) is a cost saving method. In the longer term, it enables data collection that supports more advanced use cases such as asset servitization.
How is the success of Predictive Maintenance measured for users and for the business?
In the airline industry, uptime availability, the number of truck rolls (sending out a field service technician), over the air updates, overall asset maintenance cost and time to availability.
What are the typical capabilities of Predictive Maintenance?
Some of the main capabilities are; accurate data ingestion, communication of data in near real-time (requirements can differ from real-time to daily), conversion of data into algorithms that provide input to guide decisions in reasonable lead time. Other capabilities include the amount of historical data, cleanliness of data, ingestion of new data, legacy footprint in regards to legacy protocols for data communication, data analysis location
Where is the “edge” of the Predictive Maintenance solution deployed?
A wide array of field assets – any high-value equipment that has a high downtime cost either due to loss or productivity or a resulting bottleneck. Applicable to assets ranging from medical diagnostic equipment to agriculture equipment, to wind turbines.
Deployment Challenges
What business challenges could impact Predictive Maintenance deployment?
A considerable amount of historical data must be available. In some cases high quality, useful data is readily available. In many cases, there is limited data or the data is not useful for designing the algorithm. IT capabilities should ideally be available in the customer organization but could also be outsourced.
What integration challenges could impact Predictive Maintenance deployment?
Data sharing is a significant challenge both across companies (almost impossible) and even across equipment owned by one operator due to use of different communication protocols.
What installation challenges could impact Predictive Maintenance deployment?
Deploying in process manufacturing or other facilities that are running 24/7 can be particularly challenging due to the need to install technology without disrupting operations. Green patch solutions that deploy a new system on a specific production line in a larger facility can be effective for establishing value before a large investment. Location can be a challenge in specific environments such as a deep earth mine or an offshore oil rig.
What regulatory challenges could impact Predictive Maintenance deployment?
Regulatory challenges vary greatly by industry. In medical and financial challenges can be significant. Any time data from a medical device is accessed or an external system impacts device operations there will be regulatory implications. The regulatory challenges will vary depending on the application.
Different Methods for AI to Make Predictions
Unplanned downtime reduces productivity and requires costly truck rolls to resolve. AI can predict equipment problems and make your products more reliable in the field by helping you:
- Predict with what you know: Merge your historical performance data, engineering specs, and real-time analytics to create user-specific, condition-based alarms and alerts so you can fix an issue before it occurs. This is usually the first stage of AI-enabled predictions.
- Predict with what you learn: Whether you have an established IIoT program or are newly connected, AI can help focus and refine your data to create more accurate and effective models over time. Create a predictive maintenance strategy that continuously builds knowledge and identifies triggers that predict downtime and can be proactively resolved.
- Predict with simulation: Simulate the same stress that causes performance problems during the design process to ensure machines stand up to real-world conditions and determine predictive alarm and alert points. By using data collected using AI-enabled processes, you can even improve the simulations you run over time.
Benefits of Using AI in Predictive Maintenance
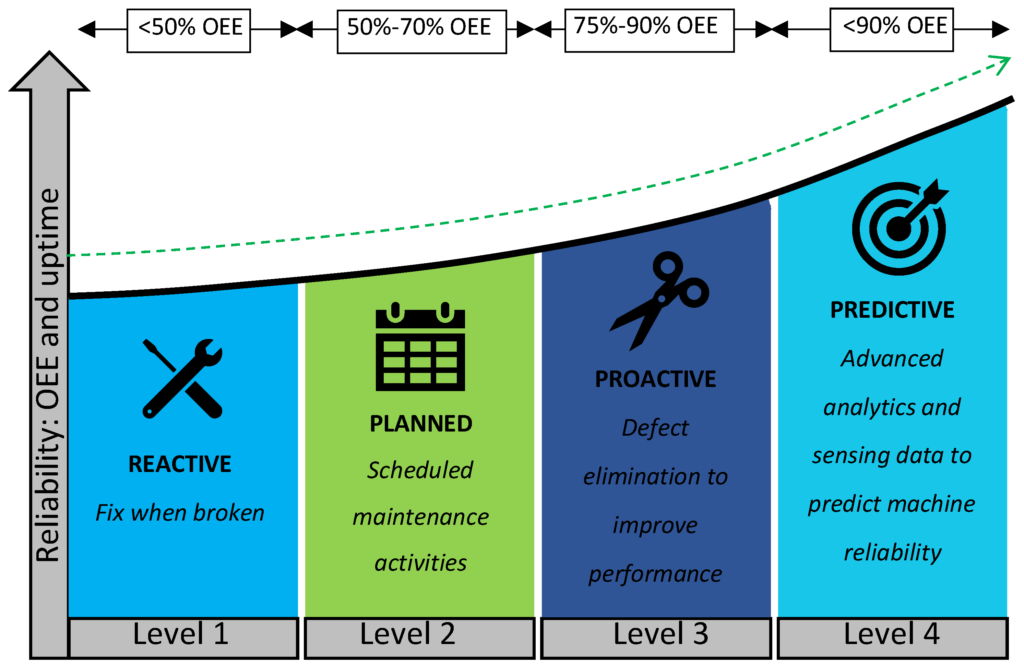
Eliminate production losses
Using AI, predictive maintenance models evaluate many variables that reflect an asset’s current status, make predictions based on usage trends, and inform maintenance teams of potential equipment failures in advance. User-specific alarms and alerts can help you prevent problems before they occur, meaning customers can reduce the need for truck rolls and respond to issues with agility. AI-fueled predictive maintenance can yield:
- A 30% drop in unplanned downtime
- 83% faster service resolutions
- 75% less time on site
Increase worker productivity
When AI is used to predict when equipment problems will occur, predictive maintenance can be planned around workers’ schedules. When workers are not disrupted due to an unexpected malfunction or regularly scheduled service visit, customers experience:
- Maximized uptime and fewer productivity lags
- Increased asset utilization
Improve worker safety
By accurately predicting when a piece of equipment might experience a malfunction or breakdown, you can avoid placing service technicians in hazardous situations. These integral predictions can ensure:
- Workers are a safe distance from machines that are likely to experience malfunctions
- Service technicians can resolve issues before they result in machines becoming dangerous
Customer Satisfaction
Additionally, because predictive maintenance can give you the data to save customers up to millions of dollars in reduced downtime, customer satisfaction will increase, leading to higher renewal rates, lower churn, and better net promoter scores. Simply put, the shift to predictive maintenance results in reduced service needs, faster and less disruptive service visits, while maximizing uptime, productivity, and safety. That’s a shift your manufacturing customers will embrace readily.
Conclusions
With AI bolstering the capabilities of predictive maintenance alongside automation, real-time analytics, and enterprise-wide connectivity through IIoT, actualizing Industry 4.0 looks promising. Even though the implementation of predictive maintenance is not without some challenges, such as an in-depth planning process, integration with current assets, and bringing staff up to speed on the new technology, organizations are continuing to accept the practice as the best way to cut costs and resolve problems faster.
Further, as the use of predictive maintenance becomes more widespread, it can help contribute to a more sustainable future by reducing the amount of energy used on the manufacturing floor, lessening the need for costly truck rolls, and increasing the useful life of equipment.
You already make predictions based on what you know. Leverage the full potential of IIoT and AI to predict using what you learn—and reap the documented ROI, increased uptime, and greater satisfaction. Deep Tech Solutions harness the power of data through IoT integration and AI adoption to effectively predict issues and prepare service leaders to address them before problems occur – ultimately decreasing downtime and increasing customer satisfaction.