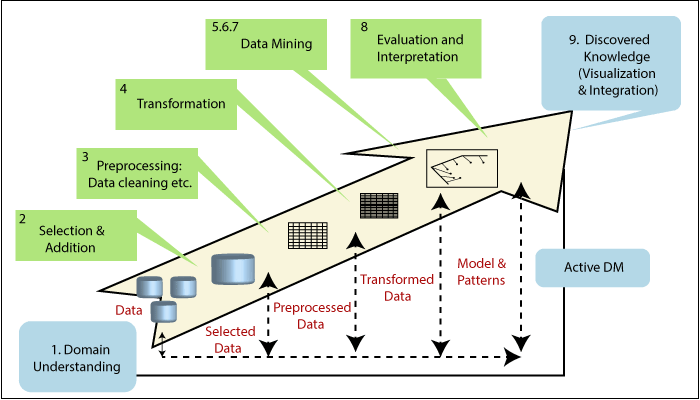
Today’s tremendous changes in Information and Communication Technology have automatically called the attention of many companies to gather copious amounts of their data on customer behavior and internal activities. Most times, they gather more data than they can efficiently make use of, in fact, in most cases, there used to be a very wide gap to better connect with customer and optimize business strategies through the data they have gathered. Industries at large can leverage this surplus of big data, by effectively combining it with machine learning to perform faster and more complex analytical tasks which goes beyond the capabilities of human beings.
No doubt, big data empowers and drives both machine learning and Artificial Intelligence (AI), since the mission of both machine learning and Artificial Intelligence is to allow computer to perform an act based on past experience inorder to take a quick decision and the experience here is in form of huge data collected overtime, the greater the amount of data available for use, the easier it will be for these systems to learn and carry out the expected function.
Data Mining
Data mining which simply refers to as the act extracting useful information from vast amounts of data, is an established discipline within the domain of Artificial Intelligence and Knowledge Engineering. It is an inter-disciplinary field, which emerges from machine learning and statistics, though, it also encompasses other areas of computer science fields. No doubt, it has received much interest from industries over some decades ago. Everyday changes in computer hardware have provided the processing power to enable large scale data mining to be conducted. Unlike other innovations in Artificial Intelligence and Knowledge Engineering, data mining can be argued to be an application rather than a mere technology which change overtime and thus can be expected to remain relevant for the future to come.
Transition from Data Mining to Knowledge Discovery
The origin of data mining can be linked back to the late 80s when the term first began to be used within the research community. During this period, there was a little agreement on what the term data mining encompassed and it can be argued that in some sense this is still the case. Data mining was known and recognized as a sub step or process within a larger process called Knowledge Discovery in Databases (KDD) during the early 1990’s.
Knowledge Discovery in Database can be referred to as the overall process of discovering relevant knowledge from data, whereas data mining refers to a particular step in this process. The Inter-disciplinary nature of Knowledge Discovery in Data (KDD) has evolved and continues to evolve from the inter-section of many research fields like statistics, machine-learning, databases, pattern recognition, Artificial Intelligence, knowledge acquisition for expert systems, data visualization, and high performance computing.
The unifying goal is extracting high-level knowledge from low-level data in the context of large data sets. The data mining, a component of Knowledge Discovery in Database relies mostly on known techniques from the field of statistics, machine learning and pattern recognition, in other to find patterns from data in the data mining step of the Knowledge Discovery in Database process.
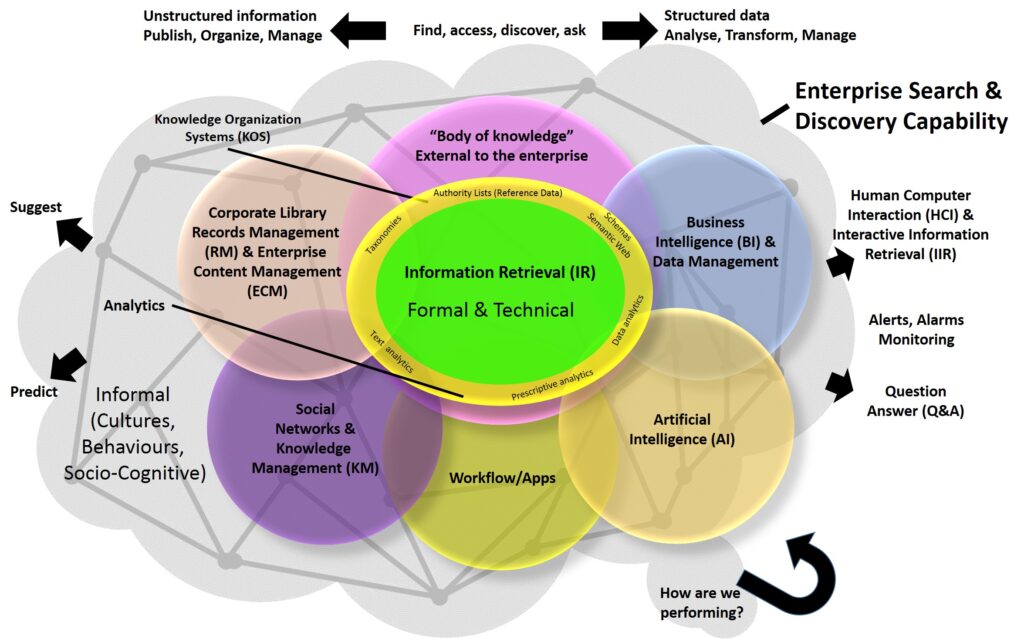
Transition from Knowledge Discovery to Artificial Intelligence
Technology is controlling the way we live, function and carrying out our daily activities. Artificial Machine in the heart can automatically fix your heart rate back to normal, while e-commerce website can recommend products for you and use computer vision to detect traffic patterns and predict accidents. No doubt, Artificial intelligence (AI) is the present and promising future of Knowledge discovery, thanks to the field of digital data pattern recognition, which is enabling software to tell humans possible outcomes and results for different scenarios.
According to the giant play maker in the field of Artificial Intelligent (Google), Artificial Intelligence can solve complex problems and has a great potential to transform entire industries, which means it is very important that Artificial Intelligent reflects a diverse range of human perspectives and needs.
Challenges Facing Artificial Intelligence
Since Artificial Intelligence are built specifically to solve a particular problem and as such they cannot be used to solve problem in other areas and many more to mention, also to develop a theoretical overview of Artificial Neural Network is not easy, likewise the extraction of information from unlabeled data (unsupervised learning), tapping into the potential of transfer learning and Scale for distributed Big data are the current challenges facing by the Artificial Intelligence.
Industrial Knowledge Discovery
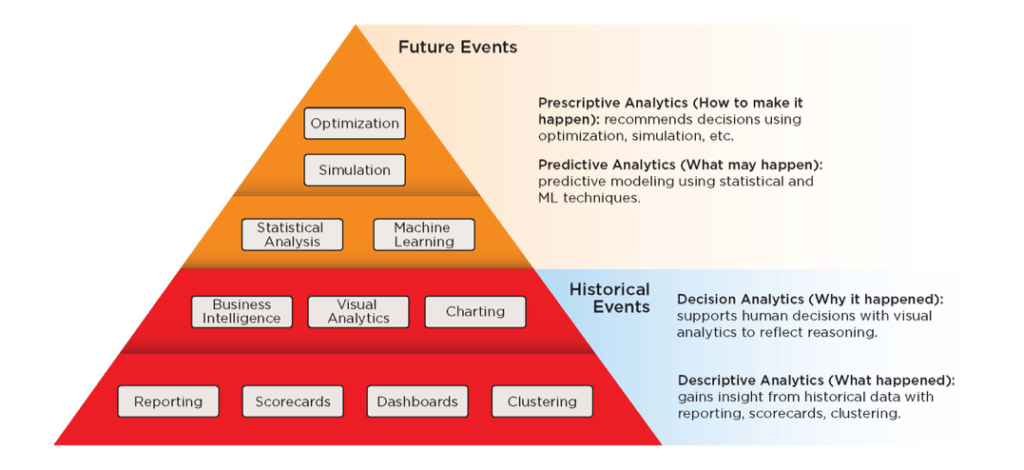
- Knowledge “lost” in shared drives and enterprise systems: Finding and accessing information storied in repositories scattered across an organization is difficult at best. Yet this knowledge is critical in an increasingly complex environment, where information from past projects is often buried in decades-old, non-integrated enterprise systems. In fact, engineers can spend 40% or more of their time searching for answers across the enterprise instead of solving problems.
- Knowledge loss due to an aging workforce: Second, in today’s world, a great portion of the workforce is 50 years of age or older and a significant portion of engineers will be eligible for retirement in the next few years. Upon retiring, these engineers will take decades of domain knowledge with them. Organizations are scrambling to address the knowledge and experience gap created by an aging workforce, layoffs and acquisitions and to quickly knowledge-enable the next generation of engineers.
Leading companies can take advantage of knowledge discovery to:
- More rapidly develop new and improved processes to retrieve and process reserves
- Reduce costs and improve operations for better ROI on capital assets, sites, and technologies
- Mitigate loss of aging workforce through capture and reuse of internal expertise
- Enhance technology solutions to stay ahead of competitors and deliver greater differentiation
- Minimize risks by ensuring compliance and not repeating past mistakes