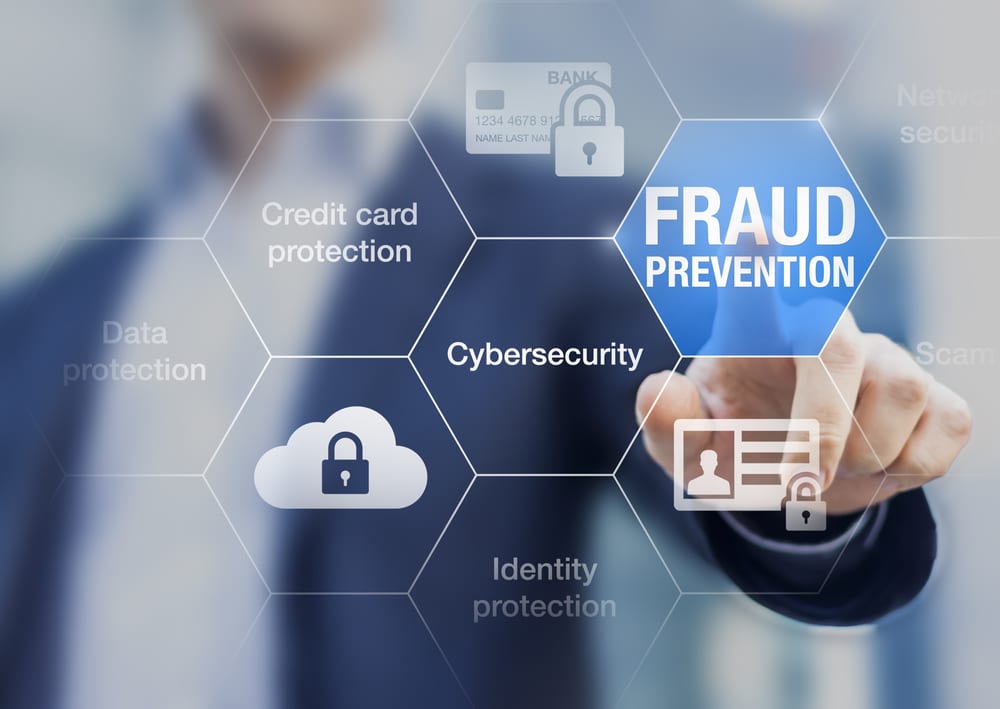
The regulatory compliance of financial institutions is growing at a faster pace than before. Moreover, technology firms are reciprocating with solutions at an even higher pace. Of late, emerging technologies like artificial intelligence and machine learning have seen practical applications, which were earlier restricted to theory. Multiple specialized and boutique firms are now offering domain specific solutions and use cases against the big players who use artificial intelligence and machine learning as a platform.
Institutions facing losses from financial crimes are growing at a similar pace. Fraudsters keep innovating and beating the system. This calls for a solution where technological advancements have to be leveraged to beat the fraudster and allow early identification of their modus operandi. The following demonstrates the key challenges in detecting fraud:
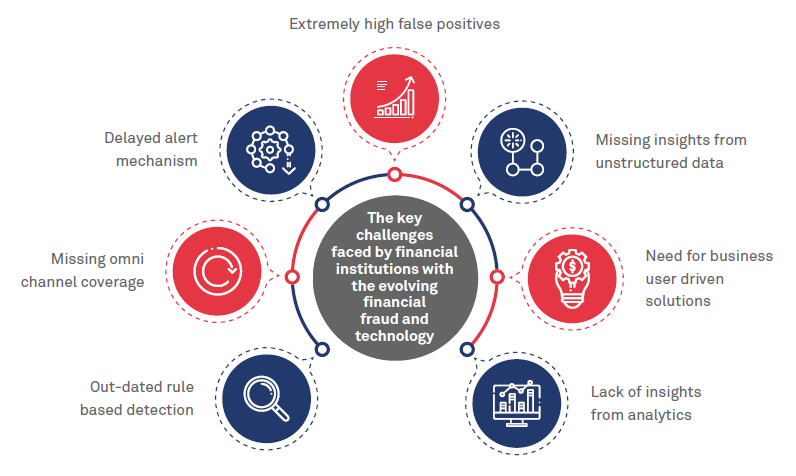
The following five drivers, sub-drivers and trends are redefining fraud detection and prevention requirements of financial institutions. With evolving technology, financial institutions are rethinking their organizations strategy to control internal and external fraud – in both offline and real-time modes.
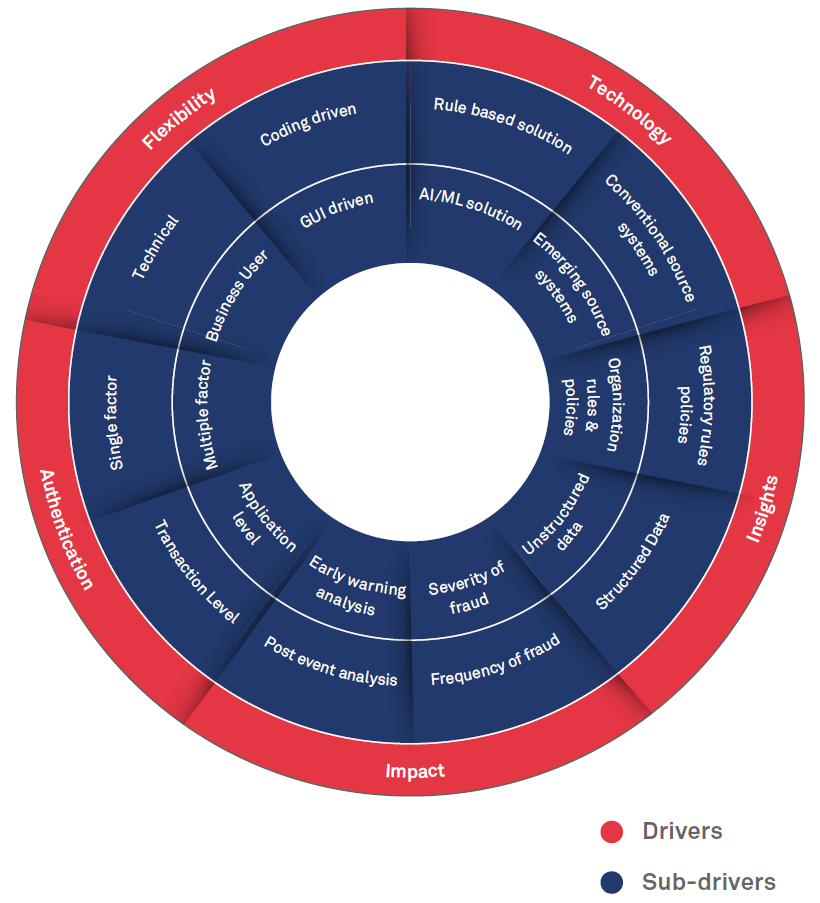
The Benefits of AI and Machine Learning for Fraud Detection
AI and machine learning can help human fraud teams maximize their efficiency in a cost-effective manner. In a 2021 publication, the FATF examined AI’s power to help firms analyze and respond to criminal threats by providing automated speed and accuracy and helping firms categorize and organize relevant risk data.
It emphasized how machine learning can detect “anomalies and outliers” and “improve data quality and analysis”. For example, deep learning algorithms within machine learning-enabled tools could perform a task repeatedly, learning from the results to make accurate decisions about future inputs. The FATF suggested several ways to implement AI and machine learning tools, including transaction monitoring and automated data reporting.
For instance, firms may be able to use AI to:
- Intuitively set fraud transaction monitoring thresholds based on an analysis of risk data. When a customer approaches or breaches an established threshold, machine learning tools may be able to decide whether to trigger a fraud alert based on what is known about the customer’s profile or financial situation.
- Detect groups of customers with characteristics indicating they’re at a higher risk of being the victims or perpetrators of fraud.
- Uncover instances of fraud in adverse media searches using natural language processing (NLP).
- Provide alert prioritization, allowing higher-risk alerts to rise to the top for review and reduce time wasted on false positives.
- Detect anomalies efficiently, going beyond individual rules to comprehensive data analysis. AI-enhanced anomaly detection pinpoints atypical or abnormal behaviors by looking at multiple weak signals that combine to identify a higher risk than they would alone.
Using AI and ML in Fraud Management: Best Practices
In 2022, the Wolfsberg Group highlighted five best practices to ensure AI and ML are used responsibly in managing financial crime risk. Each system relying on artificial intelligence should demonstrate:
- Legitimate purpose – Firms must clearly define AI tools’ scope and make a governance plan that accounts for the risk of their misuse. This includes risk assessments that account for the possibility of data misappropriation and algorithmic bias. Indeed, model governance is not new, so organizations need not start from scratch. Existing governance and model risk management frameworks can be adapted to cover AI models. They should help organizations to understand and effectively manage the specific risks relating to the use of AI.
- Proportionate use – AI and machine learning are powerful tools, but their benefit depends on the humans using them. Firms are responsible for using artificial intelligence’s power appropriately. This includes managing and regularly assessing any risks to keep them proportional to financial crime-fighting benefits like risk-based alert prioritization and detecting hidden relationships or fraud risks.
- Design and technical expertise – Because of the complexity and potential tied to AI/ML, it’s vital that teams using them – and those that oversee them – have an adequate grasp of its functions. Experts designing the technology should be able to explain and validate the technology’s outputs reasonably. This should make it possible to clearly define its objectives, grasp any limitations, and control for any drawbacks such as algorithmic bias. As per the FATF’s definition, explainability should “provide adequate understanding of how solutions work and produce their results” and is imperative for investigators’ decision-making process and adequate process documentation.
- Accountability and oversight – Governance frameworks should cover the entire lifecycle of AI and provide evidence of effective oversight and accountability. Even for vendor- or partner-provided AI, firms retain responsibility in using it as a tool. Aside from adequately training teams in the appropriate use of artificial intelligence, firms should put adequate ethical checks and balances in place to ensure the technology and its use align with their values and regulatory expectations. It’s also important for teams to understand that ultimate accountability remains with compliance officers and the firm to provide effective and compliant AML/CFT programs.
- Openness and transparency – It’s important for firms to balance regulators’ transparency expectations surrounding AI with their confidentiality requirements, especially regarding consumer data or information that could tip off potential subjects of an investigation. An ongoing dialogue with regulators and clear communication with customers can help. In keeping with this best practice, firms should also ensure the AI they use provides clear, documented reasons for its risk detection decisions. This explainability will give analysts a confident basis for continued research, ensuring a clear audit trail is in place.
Your Challenges and Our Solutions
Data Issues
- Your challenge: Digital transformation intensifies data issues. Data silos and data overload can lead to an incomplete view of risk exposures, preventing visibility of patterns and behaviors needed for prediction.
- Our solution: An end-to-end data science solution to scale analysis across your entire organization. Ability to speed modeling, training and deployment time while simplifying collaboration and adhering to a strong governance and security posture.
Predicting Fraud
- Your challenge: The rarity of fraudulent activity creates imbalanced data sets. Today’s fast transaction times and ever-evolving fraudster schemes make it increasingly difficult to immediately identify, predict, counteract and recover.
- Our solution: A platform that supports visual programming in order to upskill your team. More data science for more people, faster discovery and deployment, and deep learning and advanced analytics allow you to move from detection to prediction.
Cost of Fraud
- Your challenge: False positives require costly manual investigations. ROI is negatively impacted through loss payouts and damaged public image.
- Our solution: Make faster, more accurate decisions. Leverage unstructured data and enable deep learning and neural networks to reduce false positives. Use pre-trained API’s and one-click tooling to train and develop models faster.
Conclusions
Artificial intelligence and ML can perform certain tasks at a higher level of efficiency than even the most experienced analyst. Though some worry this might mean humans aren’t needed at all, many researchers and regulators emphasize that technology is a tool to enable and supplement human expertise rather than a replacement. Humans can still be held legally responsible for AI-informed decisions and should take steps to correct any errors that conflict with human rights. Beyond this, AI/ML can free human teams for higher-value work that’s out of reach for technology – like performing a complex investigation of high-risk activities the system has presented to them or confirming how best to action a risky alert.